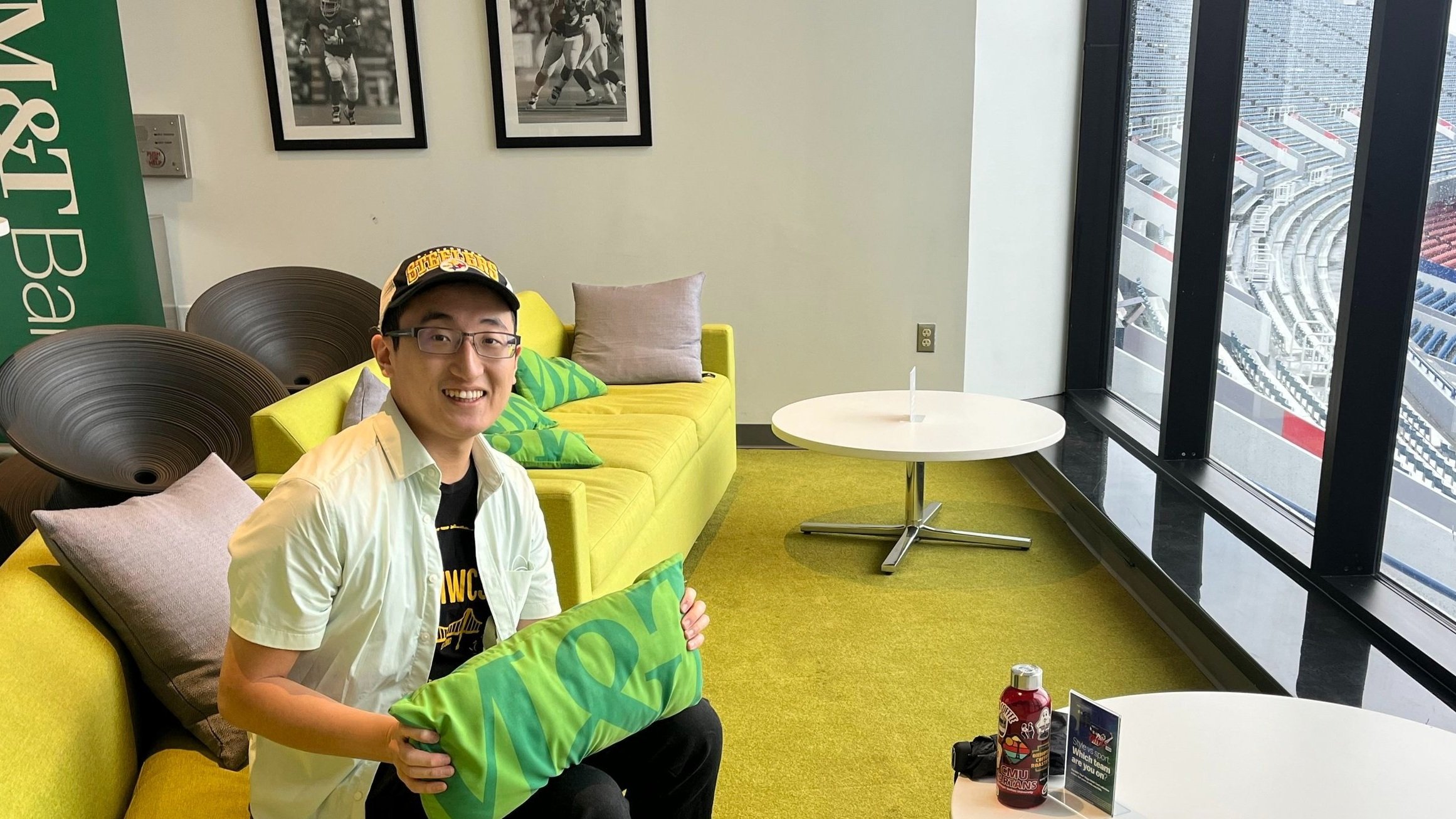
what 5Y as model risk analyst has taught me so far
I spent the past week in Buffalo, NY at my team’s annual onsite where I got to meet my entire team in person. My entire team is fully remote and scattered across the country, something I am grateful for especially with the “back to office” movement and remote opportunities to be seemingly harder to find. As such, it was a nice change to finally put the Teams/Webex screens to real faces. And I realized this would also be one of the first times gathering in person in an industry context since COVID. The last time I had significant in-person interactions in an office would be pre-COVID in 2019 when I had just started my career. This got me thinking as this year would mark my 5 years being in model risk management and model validation.
I started out my career right after graduation in model validation at a different bank where I gained exposure to an array of modeling approaches and their corresponding lines of businesses, ranging from wholesale credit and retail lending to fraud, marketing/operations, and wealth management. I worked closely with a group of highly specialized, PhD-level subject matter experts in all types of financial modeling, of which never hesitated to answer any questions I had, from “how does lending actually work?” to “what’s UPB (unpaid principal balance) or ACL (allowance for credit loss)”, or “are you sure OTTI (other than temporarily impaired) really isn’t just describing sad boy/sad girl vibes lol?” (btw, it is not, but rather a fancy accountant way to say nonperforming/defective loan)
Model validation gave me a glimpse into various different model areas that I otherwise would not have say if I had joined some other specialized team where I got to work with methodologies ranging from your traditional regression (linear, logistic, time series) to statistical risk rating scorecards or AI/ML models, as well as models internally built within the Bank or built by third party proprietary vendor of which the latter always a challenge trying to explain the role of model validation to the model owners when pushing them to get additional materials from the vendor. Don’t get me wrong though, vendor models were often interesting, such as a large vendor platform used for cash flow forecasting and liquidity management or even Microsoft Copilot using GPT-4 to be embedded into everyone’s Microsoft 365 suites.
And that’s the thing, while model risk management is largely (85%) focused on the technical aspects of the model, it is not the only aspect that is fair game for the team to provide “effective review and challenge” on in our reports or in communications with relevant stakeholders. These stakeholders include but are not limited to, the modelling team (i.e., Model Owner), line of business users, risk and control specialists across different verticals, senior leadership, compliance, Internal Audit, or even the regulators themselves! Take Microsoft Copilot for example. When implemented, the tool will leverage user inputted data across the Microsoft suite and the ChatGPT LLM engine to come up with recommendations to enhance the user’s workflow. But from the standpoint of a Bank that handles secure PII information and other confidential materials, how to ensure that this data won’t leak outside of the Bank? Similarly, who/how would be held accountable for any mistakes caused by recommendations from Copilot?
And because of the dynamic and broad (often ambiguous) sense of the work, it really trains one to have to think outside of the box, and sometimes even be creative in a seemingly quantitative and scientific field. There’s always something new to learn, even if doesn’t feel like it at times. Over the years I’ve even had the chance to gain some exposure into business reporting with managing model inventory databases and workflows in order to track model validation outcomes assessed via key performance/risk indicators.
I did not know this particular function of model risk management/model validation even existed in finance prior to starting my career, and I’ve been in the field for half a decade now. And believe me, I’m feeling existential about it too. It’s not to say I’ve any regrets starting out my career in model validation. The things I’ve learned and people I’ve connected with will forever be a part of my development. But I must admit, being a “model risk analyst” is definitely not the most sexy or prestigious position coming straight out of undergrad. Especially coming from a school where a lot of people start their careers at some boutique investment firm or software engineering, but I also realized over the years that money and responsibility are also positively correlated, such that while many of them are making 6 figures, they were also working 12-15 hour days, no real sense of work life balance. And to each their own, there are different paths and priorities, but being a model risk analyst allowed me to not only “be a quant” but also still have a life outside work where I got into ballroom and partner dancing.
I know I won’t be a model risk analyst forever, and so this feels like a nice place to reflect on my experience so far. The experiences working with cross functional teams and allowing myself to learn to get comfy in ambiguity will translate to whatever roles I do in the future!